The Marketer’s Guide to Understanding AI Agents
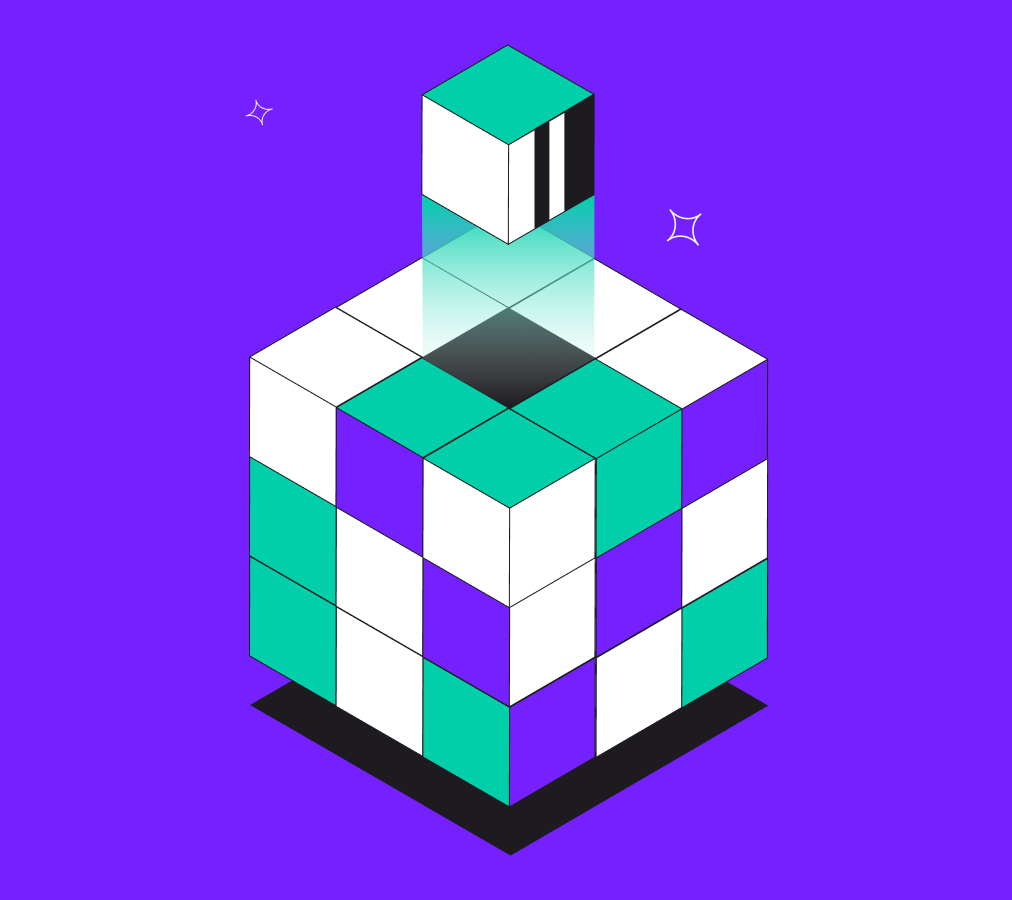
Marketers are constantly being asked to do more with less and AI is frequently pitched as the solution. But when you're not a data scientist, terms like "AI agents", "reinforcement learning," or "multi-armed bandits" can feel more confusing than helpful. If you’ve quietly opened a new tab to Google those terms, this guide is for you.
We’ll explain what they are, how they work (without the buzzwords), and what they’re actually good for when it comes to saving time, customer retention, and driving revenue in ecommerce.
To make sense of it all, we’re going to work from the ground up: starting with simple optimization models like multi-armed bandits, progressing to reinforcement learning which learns over time, explaining the predictive models Monocle built, and finally tying it all together with AI agents that orchestrate and automate the entire customer journey.
A multi-armed bandit is a smarter way to A/B test. Instead of splitting traffic evenly and waiting for the test to finish, it analyzes results in real time and shifts more traffic to the better-performing variant, while still leaving room to explore new options.
Take email subject lines, for example. In a traditional A/B test, you might send 25% of your list variant A, 25% variant B, and the remaining 50% to the winner. But what if variant A is already showing a 30% open rate, and variant B is stuck at 10%? A multi-armed bandit instantly picks up on that and starts sending more of variant A right away so you don’t waste traffic on a losing version.
Marketers typically use bandits to optimize anything where the “winner” can be spotted quickly like subject lines, popups, discounts, or SMS copy. It’s an ideal model when decisions are isolated and feedback is immediate.
Reinforcement learning is more advanced than a multi-armed bandit. While a bandit picks the best option based on what’s working right now, reinforcement learning is focused on making better decisions over time. It learns through trial and error, testing actions, observing how customers respond, and using that feedback to improve future choices.
Here’s the key difference: a multi-armed bandit doesn’t consider how today’s decision affects tomorrow’s outcome. It just wants the best immediate result. Reinforcement learning looks at the long game. It’s designed to optimize sequences, like a post-purchase journey or abandonment flow, where the timing, order, and content of messages all influence future behavior. It balances trying something new with doubling down on what works to maximize results over the entire customer lifecycle, even when results are delayed or incomplete.
Imagine a customer just made their first purchase. You could send them a welcome message, a product education email, or a cross-sell offer. A reinforcement learning model doesn’t just guess, it tests different sequences across customers, learns which combinations drive higher LTV, and continuously adapts the flow. The model learns what works best for that individual over time, not just what works best for the average customer.
The key distinction: Bandits are short-term optimizers where reinforcement learning is a long-term strategist. Both have their place, but neither on their own is enough to personalize a full customer journey.
This is where most AI marketing platforms stop but it’s where Monocle gets more interesting.
Causal and predictive models are what give our platform the ability to understand not just what happened, but why, and what’s likely to happen next. Instead of just reacting to data, these models simulate outcomes.
Predictive models also estimate the likelihood of specific behaviors, like whether a user is going to churn, return, convert with an offer, or buy again in 30 days. These probabilities feed into the AI agent’s decision-making process to choose the next best action.
Causal models go further. They help isolate impact, specifically, whether an action (like a discount) is likely to cause a behavior change. If a customer is about to receive 15% off, the model can predict whether that offer is actually needed or if they would’ve converted anyway.
Monocle’s AI doesn’t just optimize for click-through rates or open rates, it optimizes for incremental revenue. The result is smarter automation, not just “personalized” content, but actions that are tailored to the moment, the channel, and the user.
AI agents sit on top of the entire AI stack. Their job is to pull in real-time learnings from multi-armed bandits, understand long-term outcomes through reinforcement learning, and use predictive signals to tailor decisions for each individual customer. They don’t just automate tasks, they orchestrate personalized user journeys across the full customer lifecycle.
AI Agents are designed to mimic the decisions a great marketer would make, if that marketer had hundreds of hours to analyze data, test every possible outcome, and optimize every message for every customer.
And no, this doesn’t mean AI takes over your job. It just means you don’t have to babysit every email, SMS flow, or update ‘if this then that’ logic every week. Agents do the heavy lifting so you can focus on strategy.
AI in marketing is advancing quickly and so are the expectations for what marketing teams should deliver. You don’t need a technical background to take advantage of it, you just need to understand what these tools do, and how they can work together to drive better outcomes.
Multi-armed bandits, reinforcement learning, predictive models, and AI agents each play a unique role. When combined thoughtfully, they unlock smarter decisions, more personalized experiences, and more efficient use of your time.
Ready to see how this could work for your brand? Reach out to Monocle and we’ll show you how AI agents can help you make smarter, more profitable decisions across the entire customer journey.
Schedule a demo